Lenovo’s Rod Waltermann on building the hardware and software foundation for intelligent, connected, and optimized facilities
“Warehouse optimization” might be one of the least thrilling phrases in tech—at least at first glance. But throw in self-teaching computers, unparalleled camera resolution, and the kinds of innovations that could impact the storage and delivery of virtually every product on earth, and the warehouse becomes a beacon for the future. This is precisely why Lenovo is already deploying groundbreaking technology within its own supply chains and for its customers across the globe.
Warehouses are waypoints on a journey, the unsung lynchpins of a process that begins with an order and ends with the product reaching the customer. With global package volume projected to increase up to 28 percent annually through 2021, we need smarter operations—artificial intelligence is the key. Cue cameras that don’t just “see” but instead “interpret” images, and computers that stitch every bit of data into large, meaningful mosaics. Lenovo, with a product range that includes smart devices, powerful PCs, and enterprise-scale servers, is in the perfect position to drive radical transformation.
To dig into how machine learning and device advances are overhauling the concept of the dark and dusty warehouse, we sat down with Rod Waltermann, a Lenovo distinguished engineer and chief architect of cloud solutions.
What’s the need for warehouse innovation? Seems like shipping chains and suppliers already move faster than ever, at least from a consumer perspective.
Yes, things move faster, but that raises expectations, and these suppliers and their employees need to keep up. So you’re holding a hand scanner, moving a box, receiving alerts, and running through a detailed checklist. If you’re actually packing a box, say a ThinkPad with its accessories, there’s also a need to double-check that everything is tracked and packed in the right place, in the right way. You need to hire an octopus to juggle all that efficiently.
What’s the hardware component? What physical devices need to be deployed?
In the short-term, cameras are driving part of the transformation. You need a global shutter where all the pixel elements are captured at once, like a high-end DSLR Camera, to capture all the details in a rapidly moving environment—think conveyor belt. And it needs very high resolution to ensure things like bar codes and labels are legible. In practice, you’re talking between 30 high-res images every second. All this needs to be very reliable with minimal heat generation.
That’s a lot of data, righ? High-end industrial cameras like this are shooting 1800 images every minute, and each one has 12-20 megapixel resolution. You need powerful computers to store and analyze that kind of volume.
And that’s just cameras. In terms of empowering people, we have AR headsets to both capture and overlay information, and a network of connected devices automatically generating data. This is everything from stacking boxes efficiently to monitoring vibrations in a conveyor belt to predicting mechanical errors. The sensors, data, and internet of things functionality add up quickly.
Let’s jump to the software side. What connects the devices and gives them intelligence?
You have all that information coming in, but it’s not just about storage. You need a way to recognize patterns, isolate useful information, discard everything else, and send that data along for further analysis. When you’re seeking optimization and automation, the pattern recognition is essential—and that’s where machine learning comes in.
We use algorithms that recognize specific objects, deviations from expectations, or identify ways to make existing processes more efficient—and we expect those algorithms to get better at their job.
Right, like the classic example of a cat. You give an algorithm 10 images of a housecat and ask it to crawl the internet and find 1,000 more. A human then sifts through those results and teaches the algorithm why, say, 50 of those images are ocelots and not housecats. The next time it searches, only 5 are false.
Yes, but it goes even deeper. We want these programs to teach themselves, with one algorithm searching and another actively critiquing those results. This is called adversarial programming, and it’s just starting to take off.
That’s a great name. So what’s Lenovo’s role in all this?
We offer that end-to-end solution companies need. We can build out the infrastructure, from the cameras to the computers to the software making sense of it all. That means we can be scalable and customize the tech with in-house expertise. Today, companies get bits of the system independently and then set them up to work together—we’re working on sub-assemblies where only about 5-10 percent needs to be customized. That’s more efficient and, very likely, more cost effective.
The IoT trend is huge, but right now that means there are tons of startups getting into the game. It can be hard to know who to trust when you’re overhauling something as essential as warehouse operations. Fortunately, Lenovo has a clear reputation and track record of enterprise-scale success, which makes us much less of a gamble.
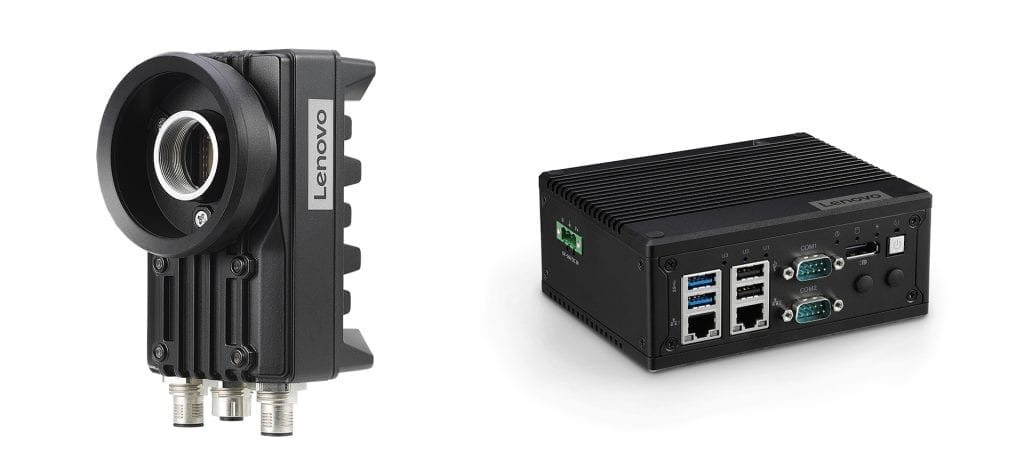
What are the bottlenecks or hurdles to seeing these innovations take off?
We can break this down into three key categories.
- Performance. You need that computing power, with high-end processors and powerful GPUs.
- Cost. Those high-res cameras with global shutters are expensive, and so are the powerful computers. Lenovo is very good at optimizing supply chains and scaling out technology, so we can mitigate a lot of that.
- Chaotic market. Some factories have been ramping up connected, intelligent operations for five years, but others are just getting started. Either way, it’s a young market space without a real leader. Remember what I said about those startups jumping in and promising new tech. Will they last? Our brand credibility helps here.
Let’s speculate a little bit. What changes will our customers see over the next decade?
AR, definitely, which we’re already starting to see tested with different partners. That’s going to help people perform better at their jobs and even change the jobs themselves. The cameras are going to get faster with even better resolutions—pushing past 50 megapixels, which will expose fine details over wider areas. Think about a conveyor belt at a pharmaceutical company, tracking more than 300 tiny pills per minute, or the bottles with small barcodes on curved surfaces racing along.
Adversarial programming will get better and the computers will be better equipped to parse all that data—those will improve hand-in-hand. And I think predictive AI will take off in the short term. We already have diagnostic trees where one little error, usually negligible, can be isolated and tracked as it develops into a more substantial issue. Or the way specific use patterns may lead to a certain part malfunctioning, and the AI sees that pattern and recommends the fix before anything even has a chance to break down. We’re already doing that sort of preemptive adjustment with software updates and patches on Lenovo systems.
Any concerns about job creation or obsolescence? As the tech gets smarter, will it limit opportunity in some way?
That’s a tough one, and it’s a balancing act we’ve seen since the Industrial Revolution. Say some technology replaces a handful of people by making repetitive, manual labor go faster. Short term, that may cause changes in the workforce, but it creates new jobs and opportunities—either around that transformational technology or elsewhere in the operation.
Take tessellation, which is the packing or configuration of different shapes in the most optimal way. Humans are still much better at this than computers, but they might work in tandem with AR overlays in the near future. That could speed up the work, increasing volume, which ends up creating new jobs or at least sustaining current numbers. Technology itself is benign, right? It depends on how you use it.
Yeah, innovation often opens many more doors than it closes, though it’s hard to predict what those will be. Anything on the horizon for Lenovo in this space?
We have some exciting partnerships in the works, testing prototypes and piloting some very intelligent warehouses and supply chains. Not much I can say just yet, but there are definitely exciting things to come.