Machine learning (ML) might be a buzzword for some people – an overused term for teaching machines to make decisions using human-like reasoning. Sure, ML might have three main data challenges. For enterprises, though, machine learning at scale helps unlock insights that save significant costs and deliver a competitive advantage. As a company with manufacturing operations around the world, we’ve seen this impact firsthand. I’d like to share with you how my team in the Digital Analytics Office is partnering with various business functions to use machine learning to help automate manufacturing in our smart factories.
The Rise of Industry 4.0
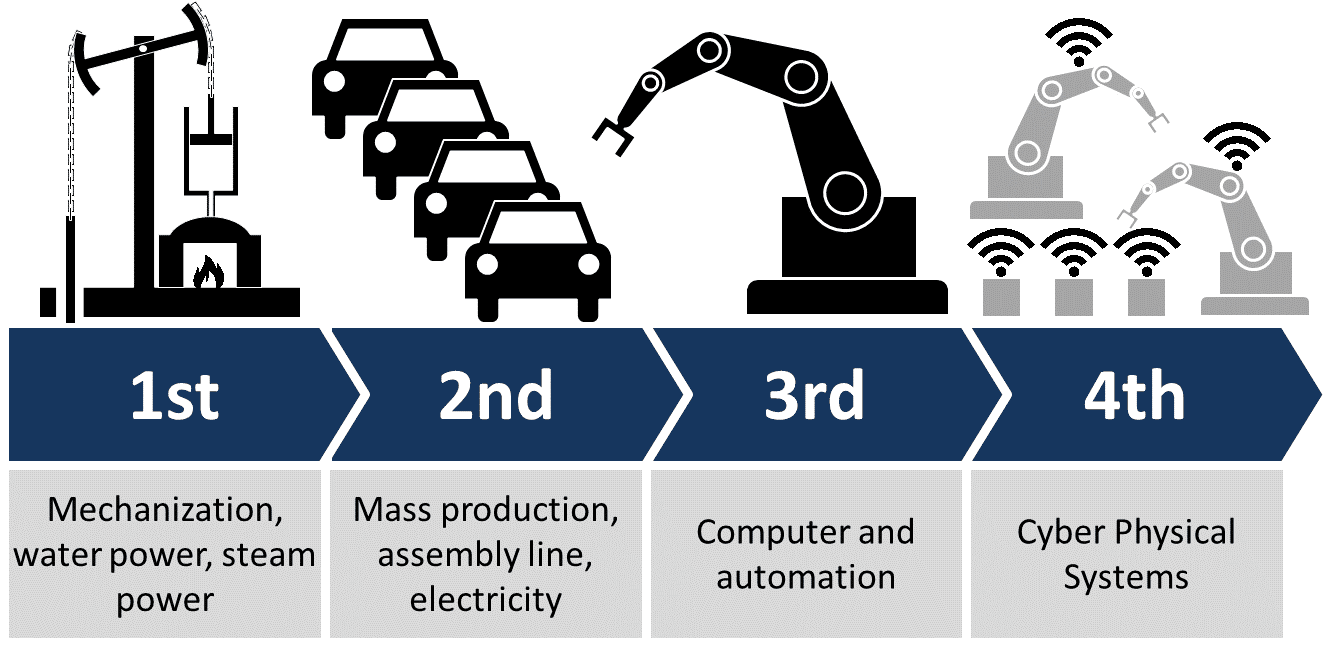
First, some context around the broader manufacturing industry trends. Right now, the industry is undergoing a fourth wave of industrial technology change known as Industry 4.0. Connected industrial devices have started to see wider adoption in many areas – from IoT gateways to networking equipment and more. In this change, companies are combining data from networks of IoT and IIoT devices and computer-controlled systems with cloud and ML computing to accelerate the journey towards fully automated smart factories.
Smart Factories Leverage Data with ML
Now, let’s go into how we fit into the picture.
We’ve used machine learning to harvest our data from millions of manufactured products and capture business value in different environments. For example, our ML model learned to automatically spot defects during the manufacturing of our wafers and drives. This modeling is critical to keeping up the high-quality and yield of our products. We predicted optimal test time for each drive to keep test times low as we push the boundaries of future hard drives to 20TB and more. With these innovations, we were able to accelerate the development and qualification of our hard disk drives (HDDs) and solid-state drives (SSDs).
Machine learning is also valuable for keeping manufacturing equipment up and running. Using sensor data from manufacturing tools, we predicted when machines are most likely to need repairs. Natural Language Processing (NLP) is being used to explore machine logs and find the shortest path for possible fixes. In addition, ML helped us reach an even stronger balance of productivity, labor, and throughput in our manufacturing operations.
To add more insight, I’ll get into three recent applications of machine learning in our smart factory initiatives.
Machine Learning Helps Improve Product Quality
Each year, we produce tens of millions of data storage devices, platforms, and systems. As you might imagine, this means a bunch of data points. For instance, we scan every sector of every track of every surface of our products for defects. We’ve found that this in-depth analysis generates over 100 trillion data points – and that’s just for a single HDD!
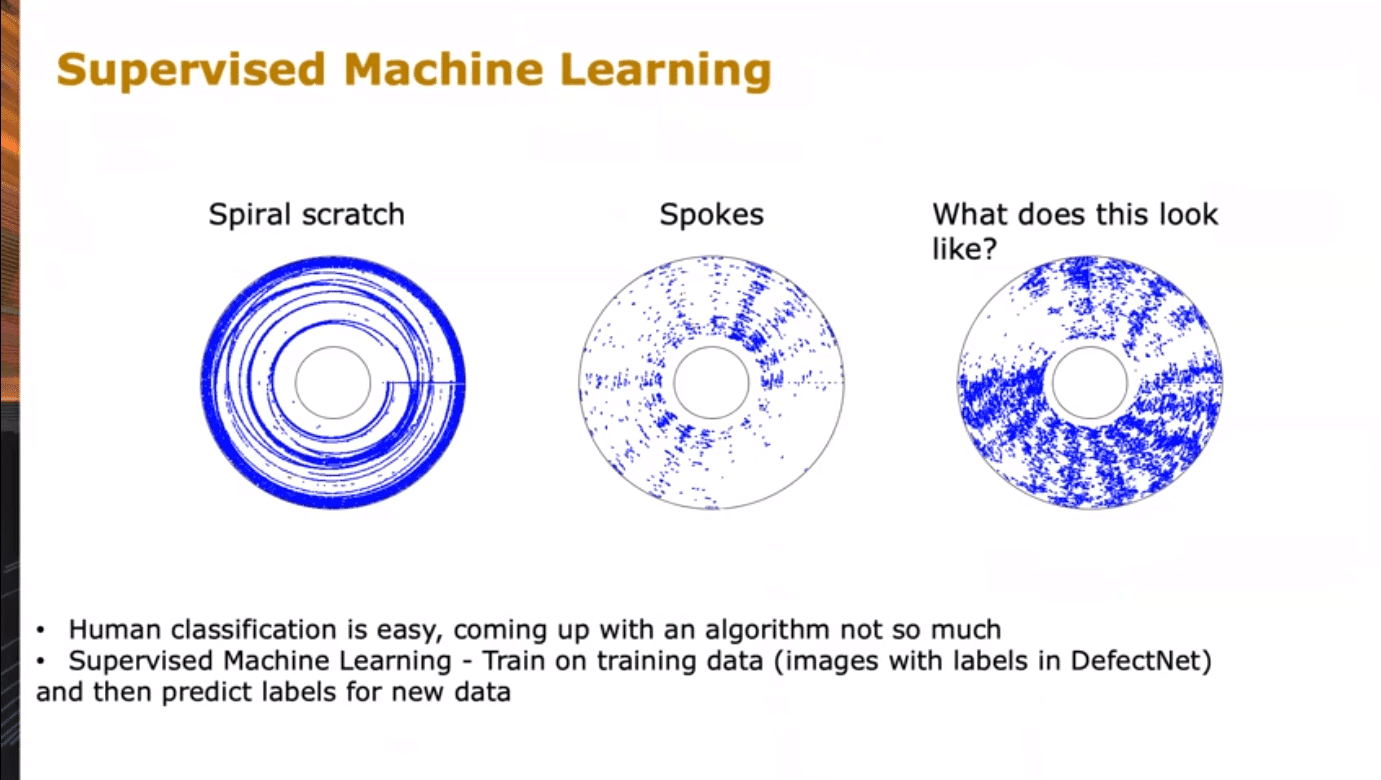
My team and I applied machine learning to two key areas in our manufacturing process: image classification and data visualization. The images being classified were defects in produced wafers and HDDs. Our innovation was actually pretty straightforward. We converted data distribution on the surface to an image, and then applied supervised machine learning to classify the images identified into distinct categories. On a typical day, our deep learning system monitored and classified over 1 million manufacturing images.
Each pattern is linked with a different root cause. For example, certain patterns can be traced back to sputter tools. Others could be related to mechanical handling of manufacturing materials. The list goes on and on. Through this method, we were able to catch warning signs of defective drives early and take them off the line before they were sent into the field. These warnings trigger both quality check and upstream process improvement to keep the chance of further defects as low as possible.
Preventing Inspection Bottlenecks in Smart Factories
We also applied machine learning in our wafer manufacturing. Wafers are critical components in the memory technology of our HDDs and SSDs. From start to finish, the process to create a wafer can take multiple months and several hundreds of steps. Historically, a large portion of these steps included a visual inspection by a technician. Using a scanning electron microscope and other inspection tools, an inspector would check the wafer for conformity and correctness – basically, any defects. This manual review process makes it nearly impossible for humans to catch every error.
Instead, we trained our ML model on a set of both defective and non-defective class of wafer images. After training, the model was able to automatically classify new inspection images and assign a probability ranking for failure with high accuracy. We thus created an automated analysis! This innovation helped reduce labor expenses, prevent manufacturing bottlenecks, and improve throughput.
Now, we’re exploring the use of upstream equipment sensor data to predict inspection outcomes. So far, our work has been scaling image labeling and classification of manufactured drives as defective or baseline. Through this method, we might be able to dynamically increase or decrease the length of inspection based on the predicted value of the outcome (defective or non-defective). The result would be a better use of inspection resources and reduction in bottlenecks.
Optimizing Work-In-Process Inventory in Factories
As I mentioned before, wafers have to go through a complex manufacturing process. At any of these steps, manufacturing tools can go down unpredictably. We needed rapid visibility into the health of our factories, down to the tool level. Having this insight helps redirect resources and production plans to meet demand in real-time.
To solve this logistical problem, we monitored the state of tools in our smart factories through a dynamic network graph. Each factory was thought of as a self-contained network of tools. Process steps were nodes in the graph. The movement of materials between process steps were represented as edges. With this dynamic visualization, our manufacturing teams were able to track the status of work in progress (WIP), pinpoint production bottlenecks and re-optimize workloads – all in real-time.
Here is a high-level view of a dynamic network graph that shows evolving work-in-progress, with process steps as nodes and material movement between steps as edges. Larger nodes suggest more WIP at that node or process and the pie chart therein shows how WIP is distributed across tools at that process step.
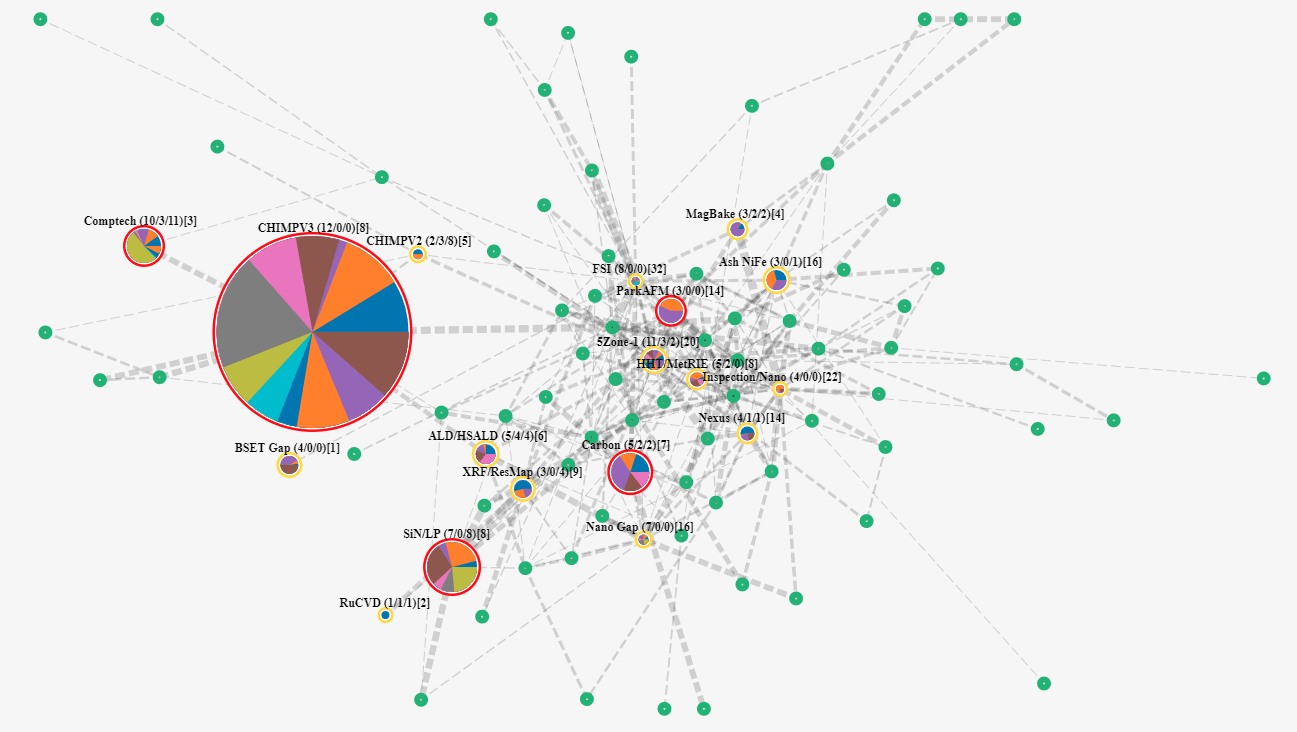
Machine Learning Moves the Bottom Line
In the end, we’re all measured on the business impact of our work. Thankfully, our rollout of machine learning is going smoothly. We’re already seeing great value. By identifying and removing bottlenecks in the manufacturing process, we saved several millions of dollars in capital expenditures. Also, we created an infrastructure that can rapidly respond to dynamically changing demand conditions – a big step towards smarter factories.
Now, we’re bringing our experience using ML to move the bottom line to our enterprise clients. Our management solution actively monitors thousands of devices in data centers, potentially saving businesses millions of dollars each year.
Learn More
Our case study explores how we’re shaping intelligence on our smart factory floors here.